TIME-VARYING EFFECT MODELING
Brief TVEM overview
Time-varying effect modeling (TVEM) allows scientists to understand the way associations between variables change over time. TVEM is an extension of linear regression that allows the association between two variables to be modeled without making assumptions about the nature of the association. For example, TVEM does not force an estimated curve to be linear.
TVEM was developed for use with intensive longitudinal data (ILD), but it has been expanded to be used with a broad variety of data types. The examples on this page use panel data and ILD, but TVEM has also been applied to cross-sectional data and historical data.
Free Software
Teach or Learn TVEM
TVEM Learning Path
Teacher’s Corner Materials for graduate-level courses
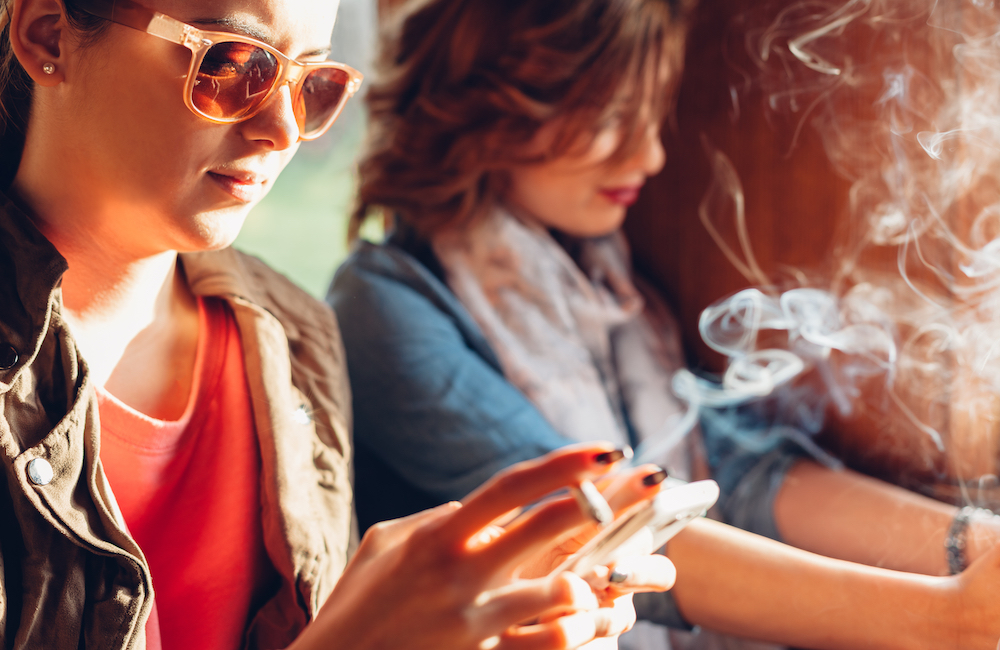
Example: Intensive Longitudinal Data and Quitting Smoking
TVEM makes it possible for scientists to observe change over time in the factors that influence an outcome. For example, attempts to quit smoking are influenced by a broad range of dynamic factors, including mood and stress level. Applying TVEM to intensive longitudinal data (ILD), we can model the changes in these associations. This allows us to determine when and under what circumstances an individual might need additional support in order to successfully quit smoking.
Read more HERE.
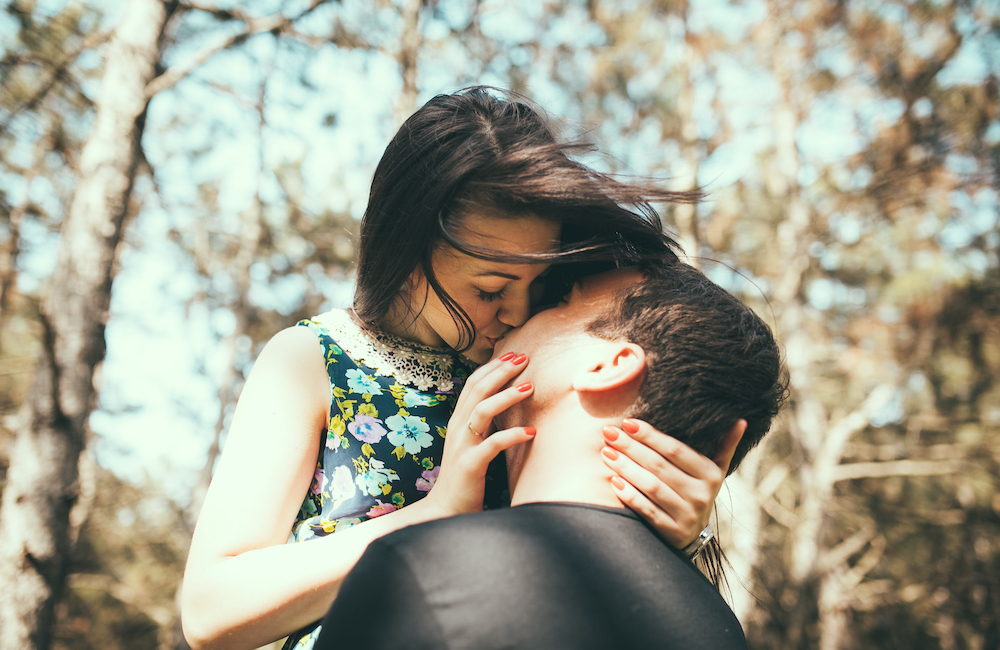
Example: Panel Data for Predicting Risky Sexual Behavior
In TVEM, time can be defined in a number of ways, such as age or time since an event. In the smoking example on the left, time is defined as days since quitting. If we consider time as age, then TVEM can be applied to panel data like the National Longitudinal Study of Adolescent to Adult Health (Add Health). In the example below, researchers use TVEM to identify changing predictors of risky sexual behavior across adolescence and early adulthood.
Read more HERE.